PHM16 Technology Demonstration
![]() |
The PHM Society invites participants to show off their diagnostic and prognostic engineering approaches through PHM technology demonstrations. The concept of the demonstrations is to offer a true “hands-on” learning experience for attendees. Multiple demonstrations will be given as brief tutorials to small groups over a two-day period. Each demo will last for approximately 30 minutes, where attendees will be encouraged to actively participate.
Demonstration Chairs |
PHM Society 2016 Technology Demonstration Attendance Sign-Up Process
All conference participants are invited to attend the interactive, hands-on technology demonstrations. There are four different demonstrations from which to choose (see below for details). Each demonstration will be presented at least twice throughout the conference. Due to limited space, attendees must sign-up in advance at the demo conference rooms on the 3rd floor (Aspen A and Aspen B). Time slots are allotted to attendees on a first-come-first-serve basis on the sign-up sheet.
Technology Demonstration Topics and Presenters:
- Machine Learning for Monitoring System Health
- PHM for Static Components
- Rapid Oil Debris Identification via ChipCHECK
- Smartphone Based Multi-Modal Sensor Fusion for PHM
Demonstration Schedule
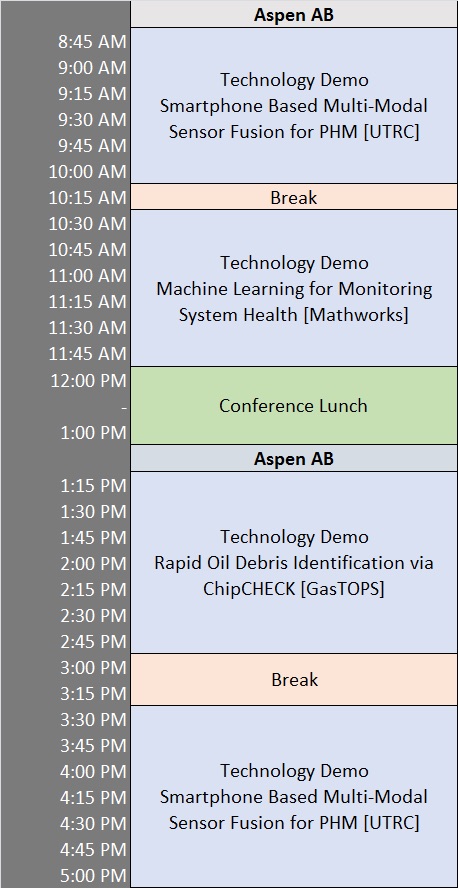
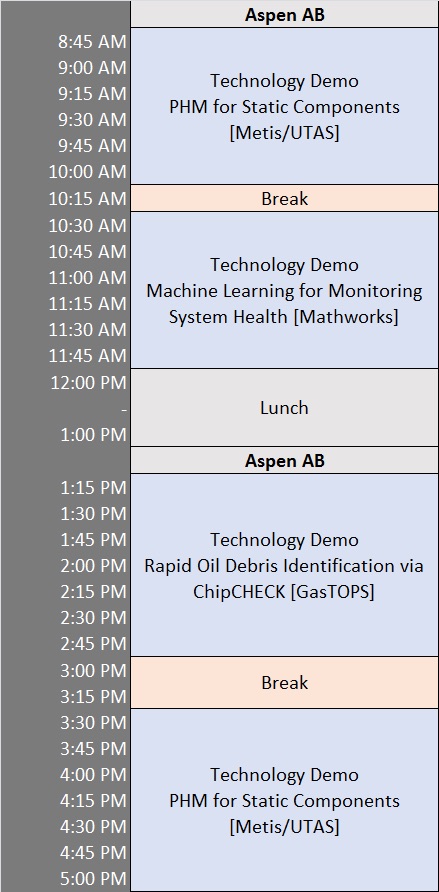
Technology Demonstration Summaries
Machine Learning for Monitoring System Health
Presenter: MathWorks |
The demo will show how you can use machine learning and system identification capabilities to monitor system health using an Arduino board connected to a machine like a DC motor.
The Arduino board will be running embedded algorithms which use the measured data from the machine in real time to determine if the system is healthy or not. The Arduino board is also capable of communicating with the cloud to store the information online where more advanced and comprehensive analytics can be run. This information can be the raw data being measured, or it can be features that have been extracted from the data using embedded algorithms running on the hardware. We will be showing how you can embed algorithms such as Support Vector Machines for classification of data as “healthy” or “faulty” and Online Parameter Estimation for creating adaptive models. The offline analysis that can be done on data being sent to the cloud can include the sending of alerts, plots that update in real time, and algorithms to run on the data, all through a web interface. Attendees will be able to interact with the Arduino board and the DC motor setup on which the embedded algorithms are running to simulate faults and failures. They will also be able to view and modify the algorithms running on the hardware setup by working with the MATLAB and Simulink models. And finally, they can view and modify the algorithms running on the cloud – via a web browser – that are accessing data from the hardware setup. |
PHM for Static Components
Presenter: Metis Design/UTC Aerospace Systems |
For aerospace applications, the most advanced fielded prognostics‐enabling systems are traditional rotorcraft Health & Usage Management Systems (HUMS). HUMS are deployed on most military—and an increasing number of commercial—rotorcraft to collect data from dynamic components to assess performance diagnostically, and to predict remaining useful life (RUL). RUL is determined empirically, typically by statistically linking vibratory frequency response characteristics to structural degradation models. While HUMS have been proven effective for dynamic components such as rotors, bearings, gearbox and other drive system elements, they have been limited to in‐service measurements of components rotating or otherwise vibrating at a known frequency. To enhance capability, United Technologies Aerospace Systems (UTAS) and Metis Design Corporation (MDC) have been collaborating on the development of next generation PHM / HUMS hardware that can also provide RUL metrics for static components (i.e. fuselage, wings, tails). These systems will function regardless of operational state.
Providing prognostic data for stationary load bearing structures will enable the extension of PHM technologies to fixed‐wing vehicles, along with component PHM, and the ability to collect data while not in operation (i.e. on the ground) will also allow for potentially simpler and less expensive hardware designed to a lower Design Assurance Level (DAL). This PHM Tech Demo will be comprised of some history and background on the techniques and challenges associated with this next generation PHM capability, culminating with a live demonstration of detecting a loosened fastener in an aluminum plate using a tablet‐based ground station connected to our latest PHM devices. |
Rapid Oil Debris Identification via ChipCHECK
Presenter: GasTOPS Inc |
Damage of oil-wetted components within engines and gearboxes are a major safety concern and a significant O&M cost driver. Correct and rapid alloy identification of oil-borne debris captured on chip detectors, filters, sump screens and magnetic plugs is critical for the maintainer to identify and assess the health of an engine or gearbox and to drive maintenance decisions. Traditional methods of assessing debris based on subjective visual inspections often lead to misdiagnosis and incorrect maintenance decisions. Off-site laboratory verification of debris introduces significant delays in the determination of equipment condition, resulting in extended unavailability of critical equipment while awaiting results. The ability to have rapid, accurate, quantitative analysis of debris by non-expert at-line maintenance personnel is not available today and represents a significant change to the current state of the art that will result in major reductions in O&M cost, improved safety and maximized availability of equipment.
GasTOPS has developed ChipCHECK, a new and innovative diagnostic tool that brings the unique capability of providing at-line maintenance personnel with immediate information on the quantity, alloy type, size, and shape of debris particles allowing them to make non-subjective, conclusive fact-based decisions regarding the health of the equipment asset and take appropriate maintenance actions. The first phase of the demonstration will include a live demonstration completed by a Gastops representative. This demonstration will include a complete tour of the software interface and a live analysis of real in-service debris particles. The purpose of this phase of the demonstration will be to allow for viewers to become familiar with the operation of the product, to observe how ease the unit is to use and how quickly results are obtained. Furthermore, this phase of the demonstration will act as the only training needed for the attendees to operate the unit. The second phase of the demonstration will provide the attendee with an opportunity for hands-on use of the unit. |
Smartphone Based Multi-Modal Sensor Fusion for PHM
Presenter: United Technologies Research Center |
Modern smartphones are equipped with multiple sensors including accelerometers, gyroscopes, barometers, magnetometers, audio and cameras. Recent advances in deep neural networks enable the creation of diagnostic and prognostics networks that can be implemented on smartphones to run in real-time and make use of as many of the smart-devices sensors as desired. In addition, many add-on sensor packs are compatible with these devices such as FLIR cameras and customized accelerometer suites. In 2015 United Technologies has demonstrated the use of smartphones for elevator PHM applications with deep learning methods. We will demonstrate this technology on a table top mechanical system. The concept is to allow participants to embed various faults to the system by changing healthy and unhealthy gears and parts. They can then start the system up with the smart-device attached or nearby and have it detect a fault and identify the cause. This technical demonstration will:
|